Data is often trapped in disparate systems (EHRs, labs, etc.), hindering the holistic view. Variations in formats and definitions impede timely insights for stakeholders. The sheer volume of data leads to overload, complicating meaningful extraction. Data quality issues like missing information and duplicates plague data and AI initiatives.
Explore strategies to optimize data and AI investments from Ochsner Health and CitiusTech. Discover how to improve patient outcomes, enable personalized medicine, predict risks, and enhance research insights.
What will viewers learn:
- Critical do’s and don’ts when implementing your data platform and readying it for quality AI output
- Key criteria’s to consider while choosing a data platform that best fits your needs
- Pragmatic examples of AI currently in production environments at health systems, their benefits and challenges encountered
- Key guiding principles on which to establish the boundaries of your roadmap for ensured success
Success Story
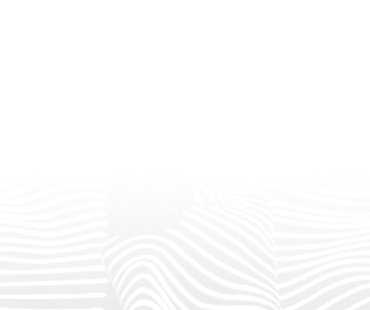
Case Study
Enhancing member engagement with cloud and real-time data
Transformed a non-profit healthcare organization by migrating to a Snowflake-based cloud data platform, modernizing care management systems, and implementing real-time data streaming. Improved member engagement and experience through unified data insights and streamlined digital services.

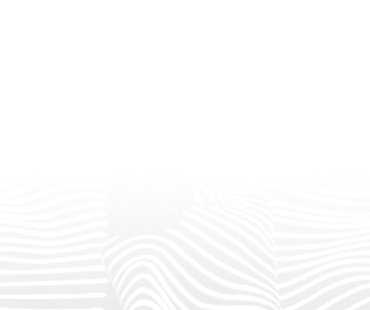
Case Study
Driving patient safety and outcomes through advanced healthcare analytics
Implemented a cloud-based data architecture for a major U.S. healthcare technology organization, utilizing Snowflake and AWS services to enable efficient data integration and analytics, reducing time to market and improving patient safety and outcomes.