- Home
- What we do
- Market
- Providers
- Quality & VBC
QUALITY & VALUE-BASED CARE
Facilitating premium value-based care with a practical price tag
Improving health and achieving sustainable financial success with value-based care
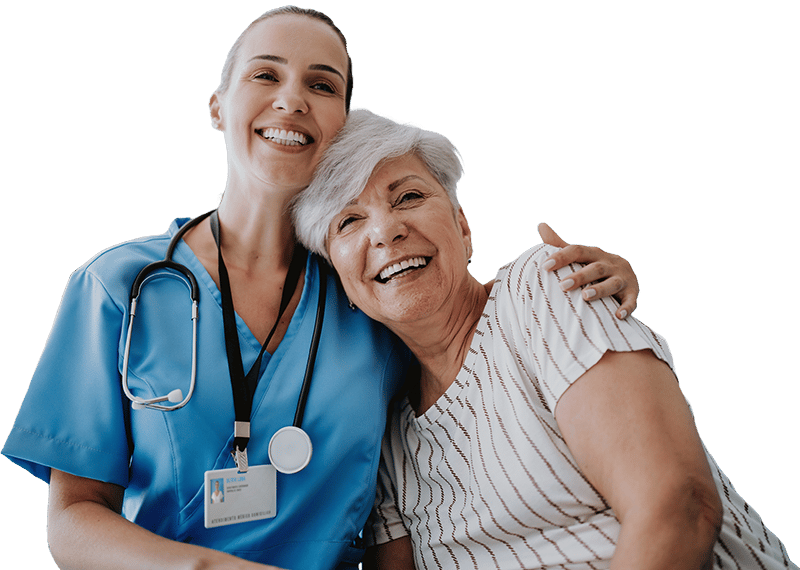
NEWS
HFS Research recognizes CitiusTech as a Market Leader in HFS Horizons: HCP Service Providers, 2024
QUALITY AND VALUE-BASED CARE
Transitioning from high risks to high returns
Pinpoint impactful opportunities to enhance healthcare outcomes and control costs. CitiusTech supports your seamless transition from volume-based to value-based care, optimizing patient care quality and financial sustainability through strategic guidance and robust technology solutions.
Insights alignment with speed of business, real-time decision support
Measure-monitor-act to proactively close care gaps
Improved quality and experience ratings
Improved utilization & efficiencies
Reduced provider abrasion and improved satisfaction
CONNECT WITH US
Analyze high-performing areas and improvement areas to improve care delivery continuously
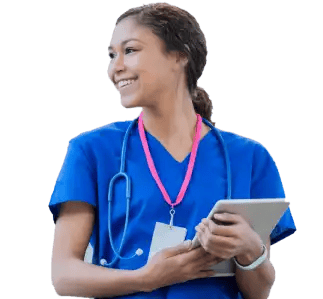
features
Meeting the unique needs with tailored solutions
Implement value-based care DataOps
Integrate advanced DataOps practices to enhance healthcare operations using Interop/FHIR, data curation, and integrated data models. Support complex data types like SDoH and imaging, all managed through modern architectures and unified governance.
Manage value-based care performance
Optimize outcomes with Perform+ suites by utilizing advanced analytics for quality, cost, experience, and risk, with regulatory reporting. Manage VBP contracts and bundled payments effectively while optimizing clinical and financial workflows.
Enhance value-based caredigital engagement
Elevate patient and caregiver interaction with innovative engagement apps including SMART on FHIR. Leverage consumer analytics to digitize care pathways and develop journey-based consumer applications, improving overall patient care experience.
SUCCESS STORIES
Solving some of the greatest challenges in Healthcare
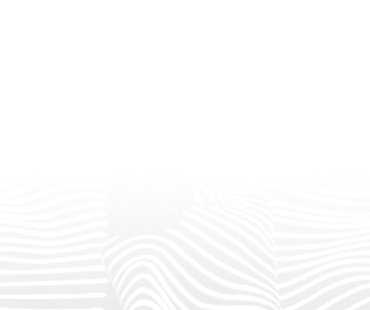
Case Study
SERVICES
Shaping Healthcare possibilities with our expertise

Digital Engineering
Reshaping Healthcare with cutting-edge technology solutions for better patient care
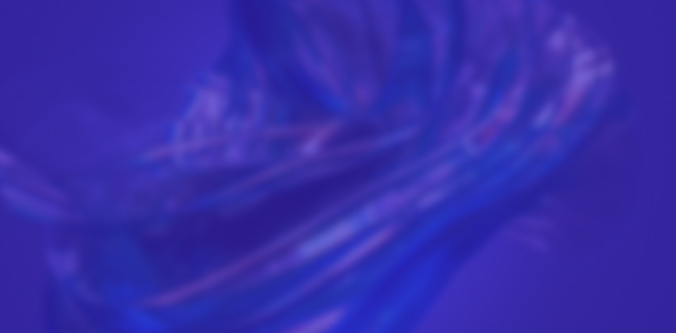
Data Engineering & Data on Cloud
Making Healthcare data the backbone of your organization's strategy
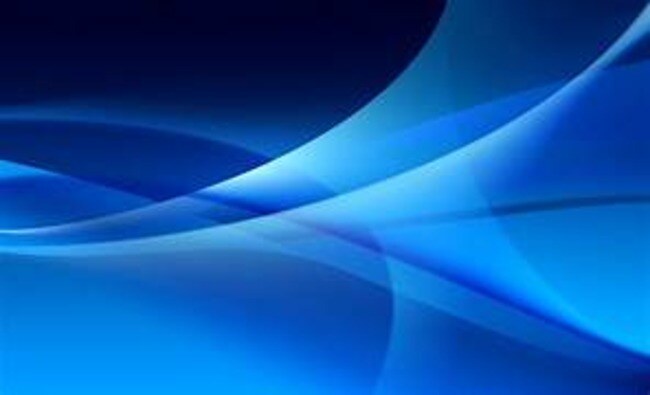


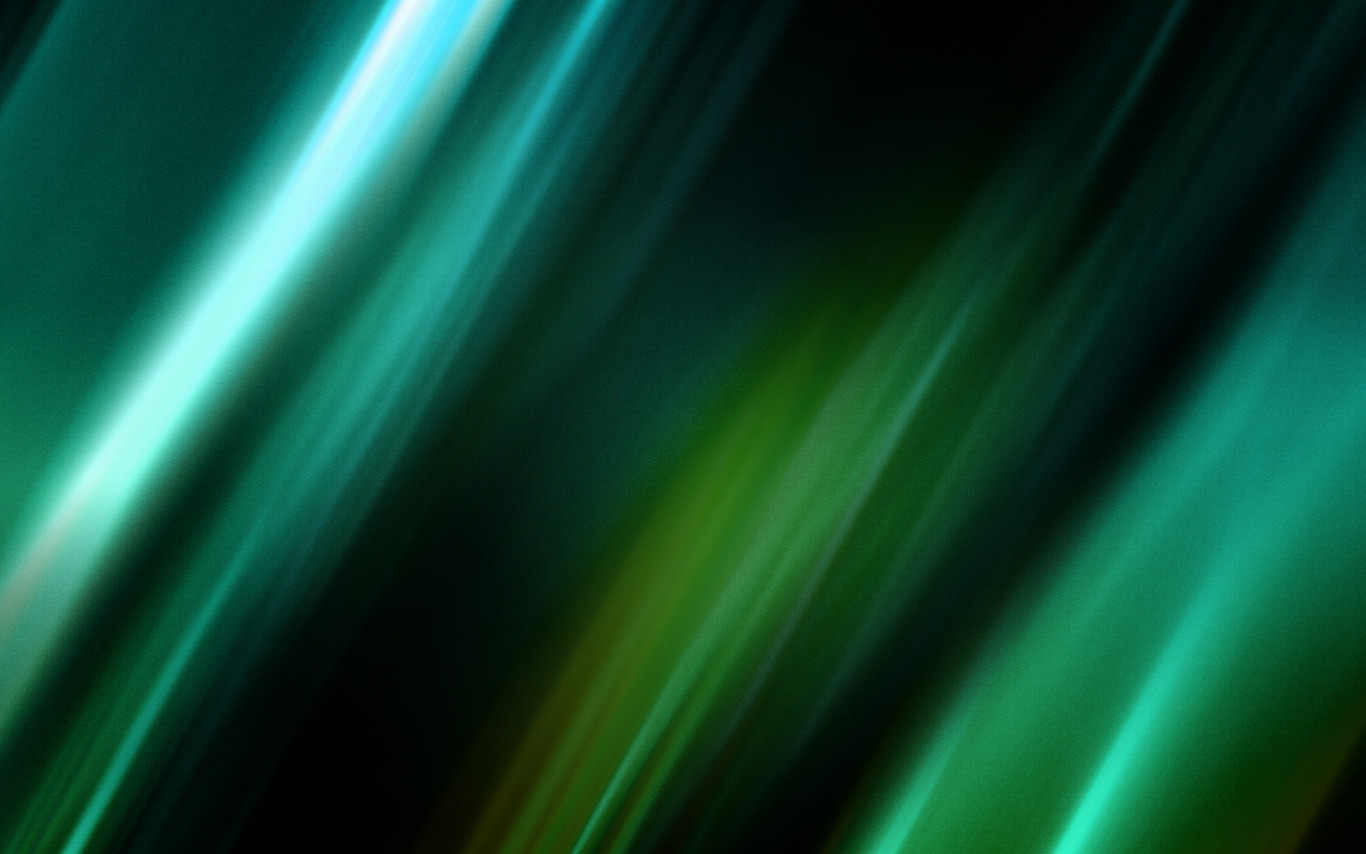
Explore other Services
Harness deep domain expertise and customized digital solutions to craft a seamless and integrated healthcare experience. With CitiusTech, you can also bridge the gap between healthcare and technology, fostering a healthier future